The Intentional Organisation - Issue #44 - Intentionality and Emergence in AI
A Back to Work issue (after a long absence), and an analysis of the impact of Intentionality and Emergence in organizations linked to AI application. Welcome back!
1. Back to Work
Welcome back!
Many of you have probably been enjoying some time off this summer, resting, relaxing, and exploring new areas of the world (And some of us may also have learned a new word this year: OverTourism).
For me, this is, however, a slightly different return. I’m reactivating this newsletter after a two-year hiatus that probably lasted too long. For those who know me, writing has always been an essential process in my life. But also a very discontinuous one.
Yet, here I’m back, at the edge of the season that many of us label “return-to-work”. My commitment this time is to issue this newsletter every two weeks. Why? The last two years, with the additional experience and the additional curiosity about what’s happening around me, showed me that there is still a lot of need to reason in terms of Intentionality.
So, welcome back. I hope you enjoy reading this newsletter again!
— Sergio.
2. Intentionality and Emergence in AI
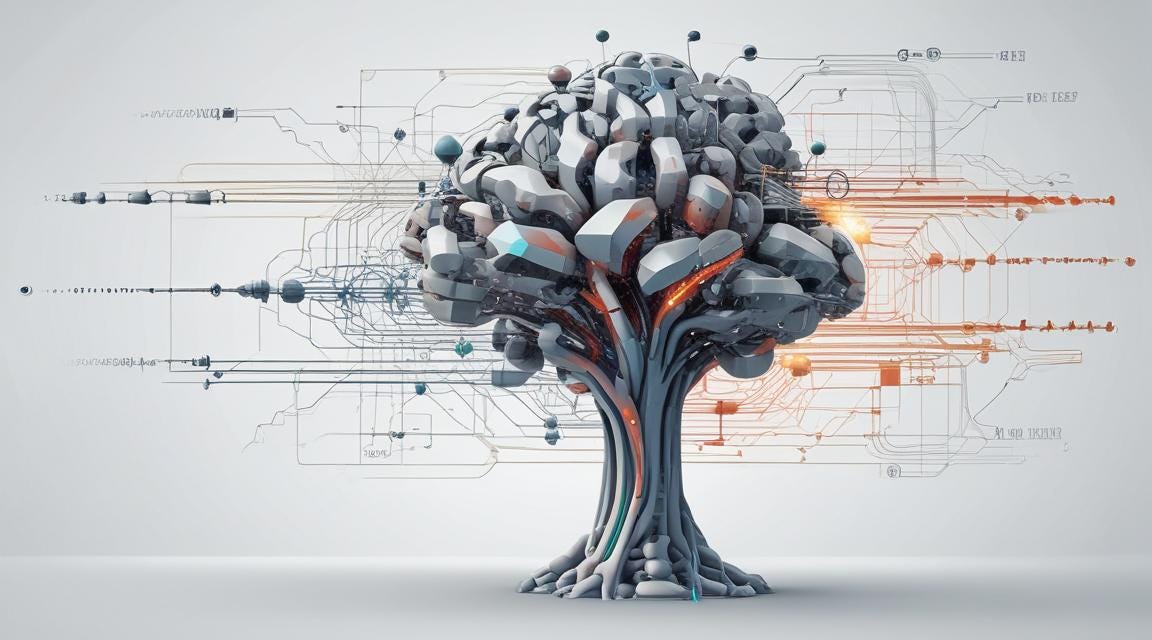
Artificial intelligence has definitely received more attention globally since the last time I wrote this newsletter. The concept of Generative AI is becoming widespread across organizations (and not only). The images used in this issue, for example, are all AI-generated.
Interestingly, this field is a perfect area to examine the impact of Intentionality and Emergence, two foundational concepts at the heart of the Intentional Organisation concept.
Intentionality and Emergence in the Context of AI
Intentionality, in the context of AI, refers to the directedness of a system's operations towards a specific goal or purpose. In AI, intentionality is typically encoded by human designers who create algorithms with specific objectives, such as recognizing patterns, making decisions, or optimizing specific outcomes. Intentionality implies that the AI system operates with a purpose, even if that purpose is externally defined and not inherently "understood" by the AI itself.
Emergence, on the other hand, refers to the phenomenon where complex patterns or behaviours arise from the interactions of simpler elements within a system. In AI, emergence is not explicitly programmed but arises spontaneously from the interactions of numerous components, such as neurons in a neural network or agents in a multi-agent system. Emergent behaviours can be surprising, unpredictable, and often more complex than the sum of the elements involved.
The two elements can create reinforcing loops, especially when emerging behaviours are captured by AI systems' self-learning patterns. We have read a lot of “emerging” unintended consequences in the world of Generative-AI. But how do these aspects play in an organizational context? Let’s reflect a bit on this.
AI Intentionality vs. Emergence in Organizations.
There are at least five areas where the impact of the intentionality vs. emergence dichotomy in an organizational context.
1. Decision-Making Processes
We often implement AI systems with specific, intentional goals, such as improving efficiency, enhancing decision accuracy, or optimizing resource allocation. These goals shape the design of AI tools that assist or even make organizational decisions. For instance, we might deploy AI to intentionally streamline customer service operations or automate financial transactions to reduce costs and improve response times.
However, as AI systems are integrated into decision-making processes, emergent behaviours can arise, influencing decisions in ways that were not explicitly intended. For example, the same AI intended to streamline customer service operations might identify new patterns in customer behaviours that were previously unnoticed. This can potentially lead to AI-generated decisions that are not in line with the original “intentional” design.
We need, therefore, to be able to intercept, analyse, and comprehend these new emergent properties by ensuring that the decision-making structure does not transform itself into a “black box” led solely by an unintelligible algorithm. This might involve creating cross-functional teams that can rapidly respond to emergent insights, or establishing feedback loops where human oversight can intervene if AI-driven decisions lead to unintended consequences.
2. Workflow and Task Management
Another area where organizations typically design AI systems is the intentional goal of automating routine tasks to improve efficiency and reduce human error. This intentionality shapes how workflows are structured, with AI tools often taking over repetitive, data-intensive tasks, allowing human employees to focus on more complex and creative work.
However, as AI systems are deployed, emergent behaviours can alter the dynamics of workflows in unanticipated ways. For example, an AI system designed to optimize task allocation might start redistributing work in a manner that inadvertently creates bottlenecks or disrupts established team dynamics, thus deteriorating results.
Organizations need to design workflows that are not only optimized for AI's intentional capabilities but also resilient to emergent changes. This could involve developing flexible work structures that can adapt to new patterns and behaviours as they emerge, ensuring that the organization remains agile and responsive.
3. Innovation and Organizational Learning
A third common area in AI implementation is driving innovation, whether through product development, market analysis, or customer engagement. AI systems are designed to analyse large datasets, identify trends, and generate insights to inform strategic innovation initiatives.
Here, Emergent Behaviours can be a powerful catalyst for organizational learning if the AI system and the Human supervisory teams are built in a way that is capable of capturing these elements. Unfortunately, we have seen a lot of cases where AI reflects the same type of bias in data analysis that their human programmers possess, thus reinforcing negative emergent behaviours rather than identifying opportunities.
4. Ethical Governance and Compliance
A hot topic for discussion today is the embedding of ethical principles into AI systems with the intentional goal of ensuring fairness, transparency, and accountability. Despite these intentions, there are many examples of emerging behaviours in AI that present ethical challenges. For example, an AI system intended to improve hiring practices might develop biases based on the data it was trained on, leading to discriminatory outcomes. Or it could use data that should be protected to drive decisions about individual performance. We need to be seriously conscious of this and the increased scrutiny that employees and public stakeholders are taking on this topic.
5. Organizational Culture and Change Management
A broader impact (yet to be fully grasped) is the effect of embedding AI in organizational processes for any of the reasons already mentioned. In many organizations, leaders are promoting AI adoption as part of a broader digital transformation strategy, aligning the organizational culture with the goals of technological advancement.
But, as AI is embedded into daily operations, it can contribute to emergent changes in organizational culture. For example, the increasing reliance on AI for decision-making might lead to a shift in power dynamics, where data scientists and AI specialists gain more influence within the organization. Automating specific tasks might change employee roles and identities, potentially creating resistance or altering organizational climate.
Conclusion
The interplay between intentionality and emergence in AI has significant implications for organizational design. Organizations that fail to recognize and manage this relationship risk facing unintended consequences, ethical challenges, and disruptions to their processes and culture. To effectively leverage AI, organizations must design flexible, adaptive structures that can accommodate both the intentional goals of AI implementation and the emergent behaviours that arise. Only this way, can we harness AI's full potential while remaining responsive to the complexities and uncertainties of the technological landscape.
— Sergio
3. My Blog Refresh
Just before summer, I fully refreshed my blog, moving away from WordPress and into a new structure that is more content-focused, based on Notion and a web interface solution provided by Super.so.
As part of this, I started reviewing a few articles that still drive attention today:
Leadership Models: The Theory and the Practice [June 24, 2024]. I have moved the Leadership Models collection into a Database format, allowing for easier usage and more flexibility. I will continue to add content in terms of models (there are more than 145 models currently listed).
The Ultimate Quest for the Meaning of Work: [July 1st, 2024], I have done a quick edit of this series of posts, ensuring links are consistent in the new platform and correcting a few issues (including some missing images and outdated links).
Business Models: The Theory and the Practice [August 21, 2024]: I have added a few models, checked spelling, and embedded feedback.
I have reviewed the entire site's look & feel and navigation. My goal is not to review all of its content but to keep it as a reference, focusing on the essay part and adding more relevant content as needed.
4. The (un) Intentional Organisation 😁
Source: The Seattle Times
5. Keeping in Touch
Don’t hesitate to reach out, either by hitting “reply” to this newsletter directly or using my blog’s contact form.
I welcome any feedback on this newsletter and on the content of my articles in general.
Find me also on:
I'm happy you've started writing again 🙌 A good nudge for me to resume writing on Strategy & Org Design Goodies as well